Modeling of parametric metamaterials with neural networks
2021/11/25
Our latest article on “Material modeling for parametric, anisotropic finite strain hyperelasticity based on machine learning with application in optimization of metamaterials” was just published in the International Journal for Numerical Methods in Engineering.
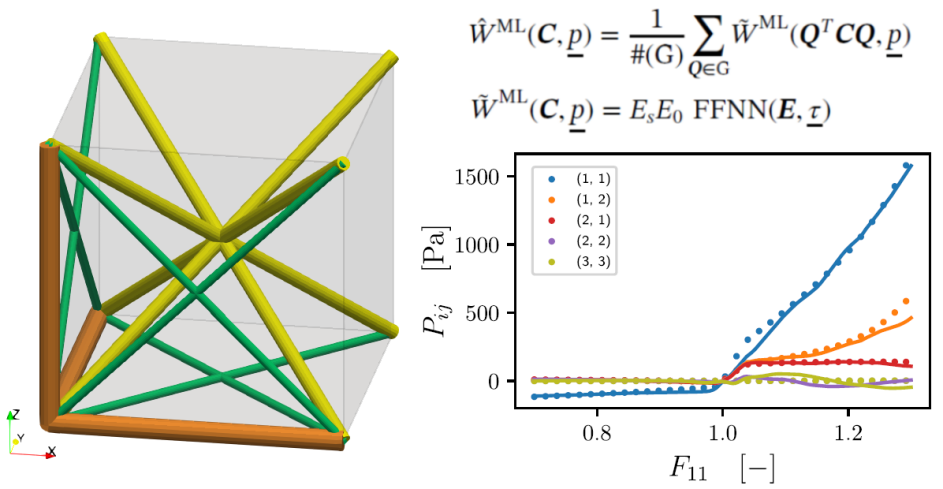
This research was led by our former Postdoc Dr. Mauricio Fernández in collaboration with Prof. Felix Fritzen from the University of Stuttgart. We extended our constitutive modeling approach using artificial neural networks to include parametric dependencies. In this way, we are able to model the effective behavior of cubic lattice metamaterials with varying topologies and morphologies over a wide range of scaled radii. Furthermore, this approach can be used for the inverse design of hyperelastic metamaterials with tailored large deformation behavior.
The article is now freely available under Open Access at http://doi.org/10.1002/nme.6869
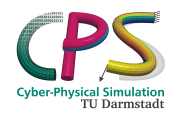