Parameterized material models with physics-augmented neural networks
2023/11/15
In our recently published article, we extend physics-augmented neural networks for hyperelastic material modeling to parameteric dependencies.
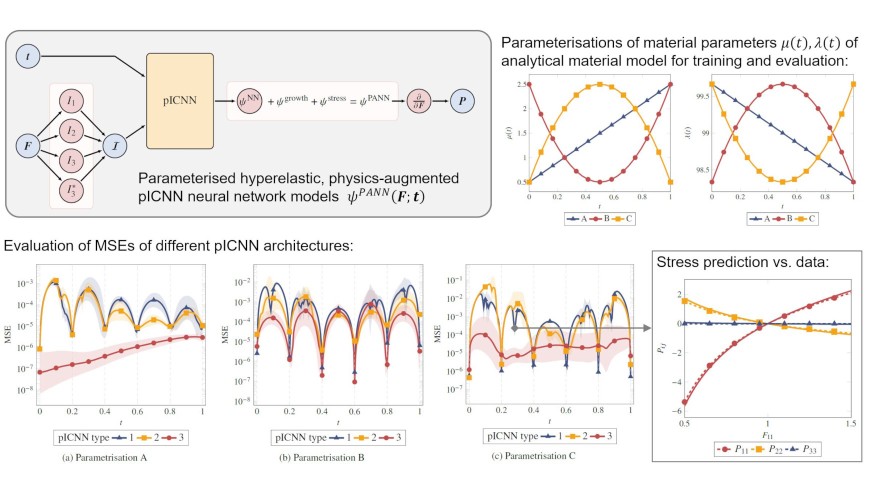
Congratulations to our doctoral researcher Dominik Klein and the student assistant Fabian Roth on the publication of the article “Parametrized polyconvex hyperelasticity with physics-augmented neural networks” in the journal “Data-Centric Engineering”!
Constitutive models relate the strain inside a material body to the stress it evokes. When describing the behavior of complex or microstructured materials, it is often desirable to include parametric dependencies into the material models, e.g., volume or aspect ratios in composites, process parameters in additive manufacturing, or microstructure characteristics of metamaterials. In this work, physics-augmented neural networks, which exploit the flexibility of machine learning but at the same time fulfill all common mechanical conditions of hyperelasticity, are extended to such parameteric dependencies. In particular, polyconvexity of the strain energy density with respect to the deformation gradient is preserved, while arbitrary (potentially also convex or monotonous) functional relationships for the parameters can be considered.
The article is available for free download (Open Access) at DOI 10.1017/dce.2023.21
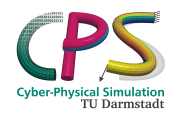